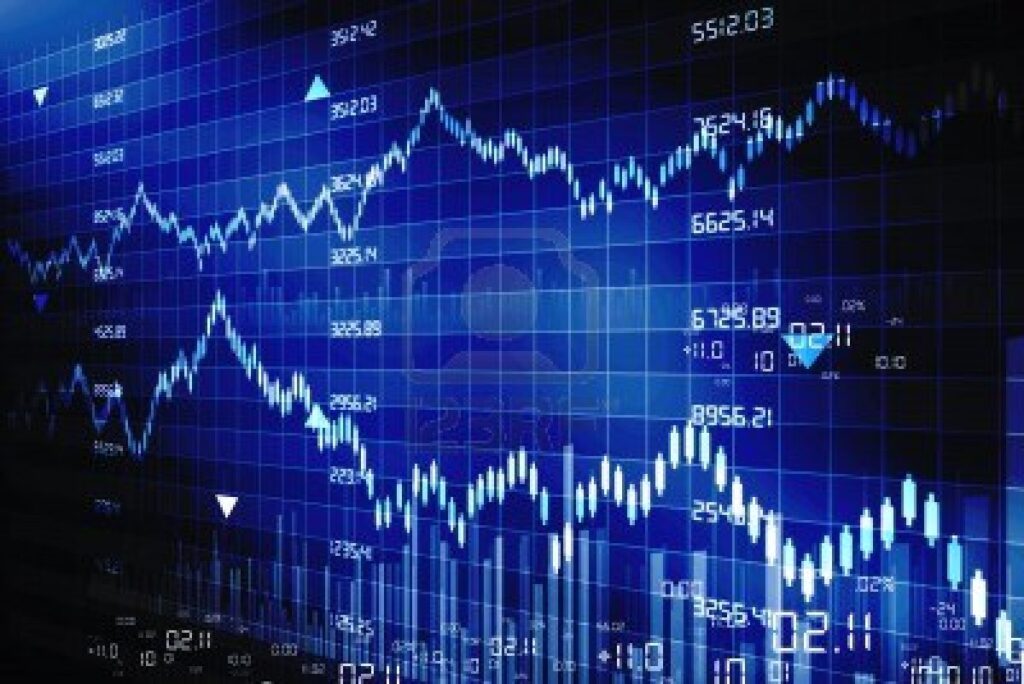
nusarayacipta.alaindonesia.com
Quantitative trading, also known as algorithmic or systematic trading, has revolutionized the financial markets. This approach to trading relies on mathematical models, statistical analysis, and computer algorithms to make trading decisions. In this article, we will explore the world of quantitative trading strategies, their benefits, and their various types.
- Understanding Quantitative Trading Strategies:
Quantitative trading strategies involve the use of complex mathematical models and statistical analysis to identify and execute trading opportunities. These strategies rely on historical data, market trends, and various indicators to develop trading algorithms. The primary goal is to remove emotional bias and human error from the decision-making process, relying on data-driven insights instead.
- Benefits of Quantitative Trading Strategies:
a) Speed and Efficiency: Quantitative trading strategies are executed automatically by computer systems, allowing for rapid analysis and quick execution of trades. This speed and efficiency are crucial in today’s fast-paced markets where every millisecond can make a difference.
b) Elimination of Emotional Bias: Human emotions, such as fear and greed, can significantly impact trading decisions. Quantitative strategies remove this emotional bias by relying on objective data analysis and predefined rules, leading to more consistent and disciplined trading.
c) Enhanced Risk Management: Quantitative trading strategies often incorporate risk management techniques that help control and mitigate potential losses. These strategies can include stop-loss orders, position-sizing algorithms, and portfolio diversification, among others.
d) Increased Market Coverage: With the help of automation and advanced computing power, quantitative strategies can scan and analyze a wide range of markets and securities simultaneously. This allows traders to identify potential opportunities across multiple markets and execute trades accordingly.
- Types of Quantitative Trading Strategies:
a) Mean Reversion: Mean reversion strategies operate on the assumption that asset prices tend to move towards their historical averages over time. These strategies identify situations where an asset’s price has deviated significantly from its average and take positions to profit from the expected price correction.
b) Momentum: Momentum strategies, on the other hand, assume that asset prices tend to continue moving in the same direction as their recent trends. These strategies identify assets that are exhibiting strong positive or negative momentum and take positions to ride the trend.
c) Statistical Arbitrage: Statistical arbitrage strategies exploit pricing discrepancies between related securities based on statistical models. These strategies identify pairs or groups of assets that have historically exhibited a high correlation but have temporarily diverged in price. Traders take positions to capitalize on the expected convergence of prices.
d) High-Frequency Trading (HFT): High-frequency trading strategies leverage sophisticated algorithms and high-speed trading infrastructure to exploit small price inefficiencies that may exist for fractions of a second. These strategies aim to profit from rapid trades executed at extremely high frequencies.
e) Event-Driven: Event-driven strategies focus on trading opportunities arising from specific events, such as corporate announcements, economic releases, or political developments. These strategies analyze the impact of events on asset prices and take positions based on the expected market reaction.
- Challenges and Risks:
While quantitative trading strategies offer numerous benefits, they also come with their own set of challenges and risks. Some key considerations include:
a) Data Quality and Availability: The success of quantitative strategies heavily relies on the quality and availability of data. Poor-quality data or inadequate historical data can lead to inaccurate models and flawed trading decisions.
b) Model Overfitting: Overfitting occurs when a model is too closely tailored to historical data, resulting in poor performance on unseen data. It is crucial to strike a balance between a model’s complexity and its ability to generalize to new market conditions.
c) Technological Infrastructure: Implementing quantitative strategies requires robust technological infrastructure, including low-latency trading systems, high-quality data feeds, and powerful computing resources. These infrastructure requirements can be costly and require continuous maintenance and upgrades.
d) Market Dynamics: Financial markets are dynamic and subject to changing conditions, including regulatory changes, economic events, and market shocks. Quantitative strategies must be able to adapt to changing market dynamics to remain effective.
Conclusion:
Quantitative trading strategies have transformed the landscape of financial markets by combining advanced mathematical models, statistical analysis, and computer algorithms. These strategies offer benefits such as speed, efficiency, risk management, and market coverage. However, they also pose challenges related to data quality, model overfitting, technological infrastructure, and market dynamics. As technology continues to advance, quantitative trading strategies are likely to play an increasingly significant role in the future of trading.